Forecasting model and maintenance
Client
The problem
Barcelona Serveis Municipals, needed a data-driven decisional tool to understand the usage and anticipate needs for parking slots users, both commercial and private, in the Barcelona Metropolitan area.
The solution
We developed an ad hoc predictive algorithm based on real-time and historic data to forecast the parking slots occupancy of Area DUM (commercial vehicles) and Area Blava (private vehicles).
We developed a forecasting model to predict the occupancy level of the loading/unloading areas in Barcelona city as well as private parking. This model was implemented using Machine Learning algorithms combining short and long-term predictions and supported by Data Analysis to ensure the correct functionality of the forecasting model.
Its purpose is to understand the impact of the variation of commercial activities in different areas and the mobility patterns of Area DUM and Area Blava users.
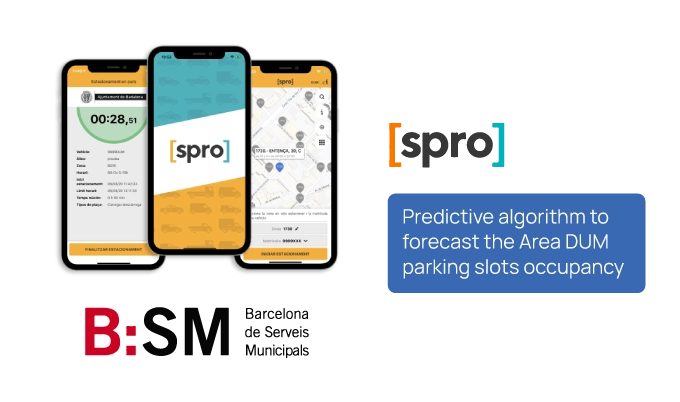
Data
From historical data on the movement of vehicles and people plus real-time data from APP usage, we implemented Data Analysis to monitor and extract the hidden insights generated by the system.
The discovery of these insights enhances the forecasting model and allows to understand the complexity of the parking and loading/unloading ecosystem.
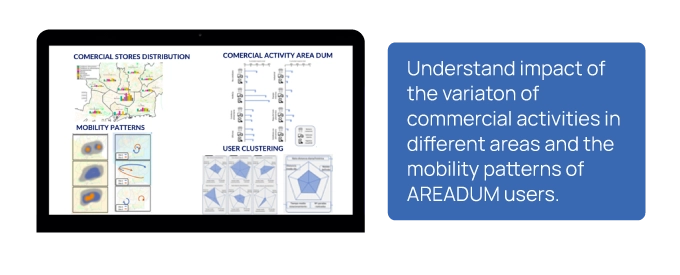
Results
Due to a successful development of the model, Mosaic Factor takes care of the supervision of the forecasting model, that previously was implemented, to assure the correct operating mode of the service.
Thanks to our software architecture and a very efficient system implementation it was possible to continuously recalculate the next two-day predictions for all the areas (more than 4.000) in less than 4 minutes.
After initial validation of the results, we maintained the solution including its iterations for the 3 years to follow.
This project has been featured by Via Empresa in an interview as a best practice in the use of data for personal mobility, automotive and logistics.