Detection and classification of disruptions’ model.
Client
Funded by the Spanish Government

Partners
The problem
Logistics networks are highly vulnerable to disruptions whose size and number are gradually increasing both locally and globally.
Despite recent efforts to improve the understanding of logistics networks and their potential setbacks, as well as technological advancements and digitisation in the field, there remains a need for a new approach or method to monitor logistics networks capable of performing a more complete and comprehensive analysis.
Our solution had to take into account the different types of factors that can disrupt logistics, including:
-
- Factors related to operations
- Weather patterns
- Natural phenomena or extreme conditions
- Political instability
- Markets instability and others.
The solution had to be scalable enough to cover entire logistics networks and incorporate all the main components of these networks
The solution
The solution of the project is a system capable to collect news, detect and classify disruption of the logistics networks, include all this information in a data lake and, finally, visualise both the news and the disruptions identified using different filters depending on customer’s needs.
The overall system architecture is shown in the figure below:
The system is composed of several interconnected components that work together to collect, process, and analyse data (news) as follows:
Data Sources: This is the foundation for generating disruptions.
-
- Bing News Search: Main news source during the initial phase.
- Scraping: Considered for the second phase, which will allow obtaining news from other relevant portals and delve deeper into them.
Disruptive system: The main system responsible for identifying, generating, and classifying disruptions based on news from data sources.
-
- Data collection: News is collected using the Bing News Search API on a daily basis, as explained in the previous section.
- Disruption Detection: The artificial intelligence model, ChatGPT, analyses the news to detect and catalog disruptions. This integration is also done through an API.
- Data Fusion: Integration of new disruptions with previously recorded ones to improve system cohesion and reliability, helping the end user to have a greater vision and understanding of the system.
- Entity Identification: Identification of locations, infrastructures, and relevant stakeholders that can help detect which disruptions are related to nodes or operators of interest to the end customer.
Artificial Intelligence model:
-
- Chat GPT: Responsible for detecting disruptions, classifying them according to their type and associated topics, and associating them with previous disruptions.
Data Lake:
-
- Database: Central repository for storing data before and after processing. This means storing both news and generated disruptions.
Frontend:
-
- Retriever: Graphical interface that connects to the database through an API and is responsible for displaying disruptions and their related news and entities. It also allows sorting and filtering news by type, first and last detection dates, and status, as well as by the various entities to which the disruption is associated.
Data
The data lake has been designed as a repository to collect potentially relevant digital news from any source, in order to process and analyse them for disruptions. During this initial phase, Bing News Search was used as the data source. The following are the different attributes that exist in the data lake, distinguishing between raw data, i.e., initial data obtained through Bing News search, and data processed by the disruption detection and classification model.
Results
Phase 2 of this project is going to be presented to the Spanish government. Here it is how the interface looks like at the moment:
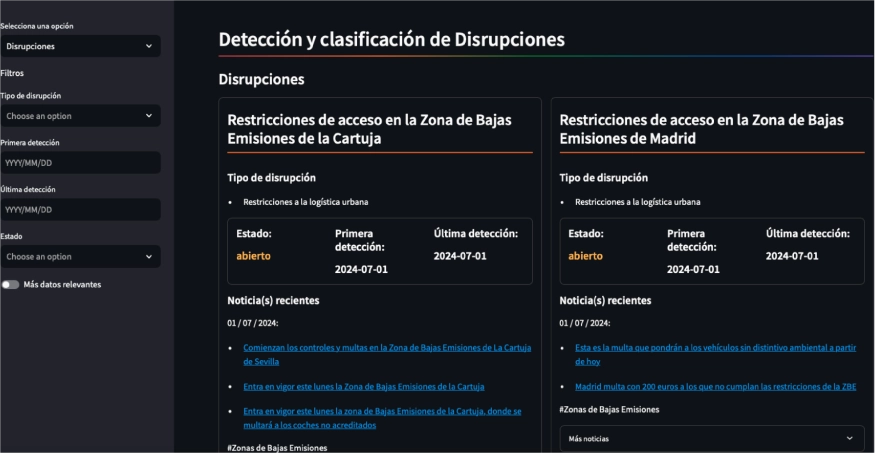
The disruptive system can be visualised live through this link: http://disruptive-retriever-fe-prod.apps.mosaicfactor.es